This blog focuses on the role of AI in Supply Chain Due Diligence and discusses both the challenges, limitations and benefits of AI within a holistic due diligence process as well as how it may evolve in the future.
There has been much discussion and debate about the use of AI in supply chain due diligence. References to terms like Internet of Things (IoT), big data, web scraping and inferred relationships have gained increasing popularity in the media and in discussions regarding supply chain due diligence. Society is increasingly harnessing the power of the internet and technological developments to change the way we live and work. Debate is widespread, as is the confusion around the capabilities of emerging technologies and their ability to support sustainable procurement practices.
The race to net zero across all industry sectors is creating unprecedented competition for much needed raw materials, expertise, sub-contractors and suppliers. This increasing demand is having environmental and social implications. There are instances of forced labour linked to solar panel manufacturing, Amazon deforestation linked to wind turbine manufacturing and marine and fish stock damage in Indonesia from illegal nickel mining.
It’s undeniable that new AI technologies like adverse media screening and satellite image analysis play a role in our ability to protect people and the planet, but when dealing with complex and often hidden issues within the supply chain, is it enough to rely on these developments?
What is AI?
First, let’s just make sure we all understand what we mean by AI. There are a number of different types of AI. These terms refer to different concepts within the field of artificial intelligence. Perhaps unhelpfully, these are often confused or used interchangeably – in particular Narrow AI, Generative AI, and General AI which are explained below.
Narrow AI (or Weak AI): This refers to AI systems designed and trained for a specific task or a narrow set of tasks. Most AI applications in use today, such as voice assistants like Siri or Alexa, image recognition systems, and recommendation algorithms, fall into the category of narrow AI.
Generative AI: Generative AI typically refers to a subset of artificial intelligence that focuses on creating new content or data rather than just analyzing or classifying existing information. It often involves models that can generate realistic and novel outputs, such as images, text, or even entire scenarios, based on the patterns and information they have learned from training data. Examples of generative AI include text generators like Chat GPT-3, image generators, and other models capable of creating new content.
General AI (Artificial General Intelligence or AGI): General AI, on the other hand, refers to a type of artificial intelligence that possesses the ability to understand, learn, and apply knowledge across a wide range of tasks, similar to human intelligence. AGI would be capable of performing any intellectual task that a human being can, adapting to new and unfamiliar situations, and demonstrating a broad understanding of various domains.
Unlike Generative and Narrow AI, which are designed for specific tasks, AGI is hypothetical and currently does not exist. Achieving AGI remains a long-term goal in the field of AI research so, for now, this blog focuses on Narrow and Generative AI.
AI Challenges
While Generative and Narrow AI can offer significant benefits in improving efficiency, accuracy, and decision-making during supply chain due diligence, there are also several challenges and issues associated with its implementation.
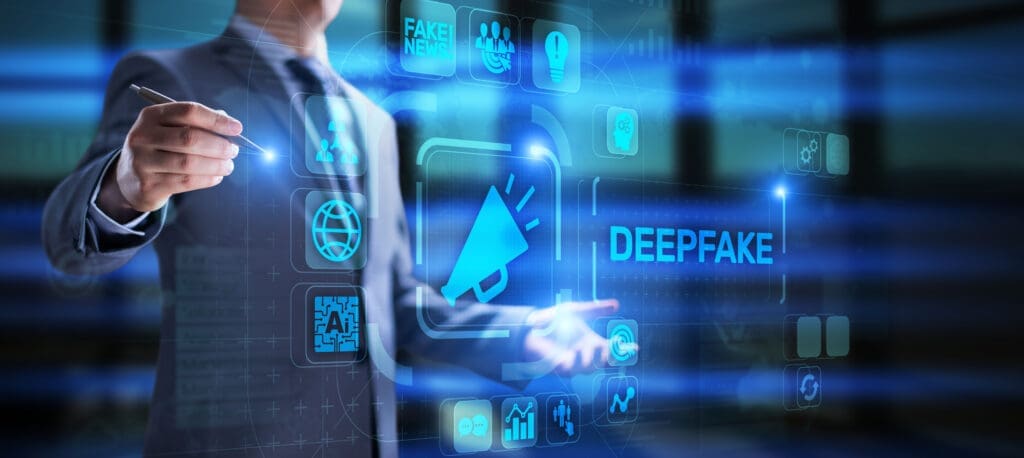
Few unscrupulous operators employing forced labour, mining illegally or polluting seas and rivers will put information about their business activities where today’s Generative AI can find it. Some of the key issues include:
Data Quality and Reliability: Generative AI systems heavily rely on data for training and decision-making. If the input data is inaccurate, incomplete, or biased, it can lead to flawed results and decision making.
Data Privacy and Security: Supply chain data often includes sensitive information about companies, suppliers, and customers. Ensuring the privacy and security of this data is crucial to prevent unauthorized access, breaches, or misuse.
Complexity of Supply Chain Networks: Supply chains can be intricate and involve numerous stakeholders, making it challenging to model and analyze. Generative AI algorithms may struggle to capture the full complexity of global supply chain networks.
Lack of Standardization: The absence of standardized data formats and protocols across the supply chain can hinder the integration and interoperability of AI systems. Different companies may use different systems, making it challenging to create a universal solution.
Interconnected Risks: Supply chain risks are often interconnected, and Generative AI may struggle to analyze and predict cascading effects. For instance, a disruption in one part of the supply chain may have ripple effects throughout the entire network.
Ethical Concerns: The use of AI in supply chain due diligence raises ethical concerns, such as algorithmic bias and the potential for unintended consequences as evidenced by Google’s Gemini AI recently. AI systems may inadvertently perpetuate existing biases or make decisions that have negative social or environmental impacts.
Dynamic Nature of Supply Chains: Supply chains are dynamic, with constant changes in demand, supply, regulations, and geopolitical factors. AI systems may struggle to adapt quickly to these changes, leading to outdated or inaccurate insights.
Validity of Time Sensitive Data: Certain AI supply chain due diligence processes rely on the collection of publicly available company data that may be contained within annual financial or sustainability reports. Due to the retrospective reporting cycle of companies that disclose this information, it can often be out of date or irrelevant by the time it has been collected and analyzed.
Accessing Lower Tier Information: Many suppliers within the complex world of a company’s supply chain do not publish or disclose information publicly. Often, micro and small businesses retain information in hard copy, or on their company servers making it almost impossible for AI solutions to access the information.
The beneficial role of Generative AI
Despite these challenges, AI can and will increasingly play a role in enhancing supply chain due diligence and risk-based thinking when implemented thoughtfully. It’s already in use day to day and will continue to evolve to include:
Data Quality Assurance: Prioritize data quality by implementing data validation and cleansing processes. Use AI algorithms to identify and rectify inconsistencies, errors, and outliers in the data.
Advanced Analytics for Network Complexity: Employment of advanced analytics and machine learning algorithms to model and analyze complex supply chain networks. These tools help to provide insights into dependencies, vulnerabilities, and potential risks across the entire network.
Regulatory Compliance Monitoring: AI tools can and are being used to monitor and ensure compliance with relevant regulations.
Integrated Risk Management: AI-driven integrated risk management systems that consider interconnected risks. This can involve simulating and analyzing various scenarios to identify potential cascading effects and develop proactive mitigation strategies.
Supply Chain Visibility and Traceability: Leverage AI technologies such as blockchain and IoT (Internet of Things) to enhance supply chain visibility and traceability. This can improve the accuracy of data and provide real-time insights into the movement of goods throughout the supply chain.
These use cases will only increase as technology becomes more mature and established. For example, Adaptive AI or AI that can adapt to the dynamic nature of supply chains with continuous learning mechanisms, ultimately, will allow for evolution and updating based on changes in demand, supply, and external factors.
AI and web scraping for SME supply chain management
Today, for SMEs, fully automated and web scraping type solutions can provide a cost-effective supply chain management solution – particularly where social and environmental risks, compliance requirements, and supply chain complexity are lower.
AI’s role in high risk, complex sectors
In the heavily regulated, complex, and high-risk industry sectors that Achilles specialises in supporting, however, it’s hard to see a scenario where AI alone will provide the transparency required – for a considerable time in the future, or perhaps ever.
Here, AI’s role, for now, is and is going to be as a supplement to deeper, more detailed methods. These are perhaps not as trendy as AI. They use real people, eyes, ears and many years of international experience and real understanding of the complex issues at hand to identify threats that lurk in the shadows of today’s supply chain.
Real world examples
At Achilles we’re fortunate to support clients at all stages of their due diligence journey, from risk evaluation to disclosure. Part of that process are our on-site audits and worker interviews. These are “boots on the ground” type activities, in some of the most inaccessible and demanding places on the planet. Our teams of Auditors use their eyes, ears and vast experience to go even deeper.
A recent example of this is the potential cases of forced labour identified at a site in Singapore. Our client, a multinational energy company unaware that migrant workers employed via an agency were being asked to pay huge deposits to gain employment and, in some cases, had their identity documents taken from them. Sadly this isn’t an isolated case. Whether its chemicals being deposited into watercourses or wage deductions as a form of financial punishment, our teams see and hear these issues with their own eyes and ears.
Today, to apply effective due diligence and to comply with ever more rigorous due diligence legislation like Apenhetsloven, Bill S211, BRSR Core, CSDDD, Lieferkettengesetz, and The Modern Slavery Act requires a broad approach using all of the tools available to a business. Fortunately for our clients we’ve harnessed these tools and have the experience to know when and how to deploy them.
Achieving a balance between automation and human expertise is crucial. Over-reliance on AI without human oversight can and will lead to blind spots and missed nuances, while insufficient automation may result in inefficiencies. At a time when the world is facing an increase in human rights abuses, child labour, forced labour and environmental pollution and fighting to reduce emissions and address climate change, we need to use all the tools at our disposal to create transparency and address the adverse impacts of our business dealings. Regulators, investors and customers would be wrong to accept anything less.